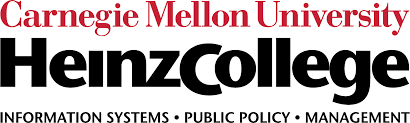
Project Overview
Over the course of economic history, one particular model for macroeconomic growth has stood the test of time: Solow Growth Model. This model, based on a Cobb-Douglas production function factoring in labor and physical capital, is being challenged as Artificial Intelligence emerges as sharing characteristics of both.
As such, Economists have been looking into different, non-linear approaches to modeling the macroeconomy at large and determining the return on investment and labor market impacts of continued investment by firms into AI.
In this paid research assistantship I assisted Dr. Anand Rao in taking economic models, such as those from Daron Acemoglu, and experimenting with them in the context of Agent-based Computational Economic models.
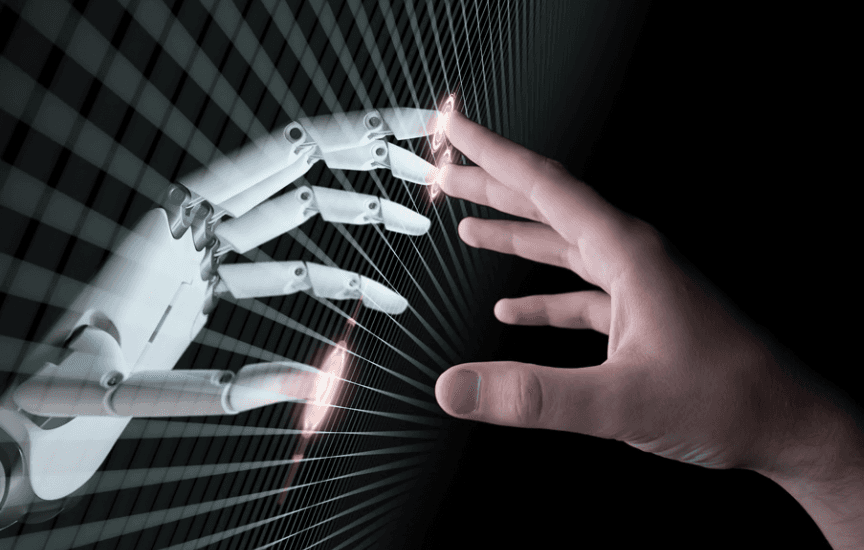
What I Learned:
What is an Agent-based Computational Economic model?
Agent-based computational economic models are similar conceptually to a petri dish experiment. Leveraging the power of various machine learning algorithms and models, economists can now define and provide attributes to agents within the model. These attributes and parameters dictate how the agents will behave in the model. Adjusting them, as well as their goals, can get the agents to behave like economic actors in the real-world, such as regulatory agencies, service firms, manufacturers and consumers.
This new-age approach to economic modeling has advanced the field of economic research tremendously, enabling more dynamic models to exist in order to consider multiple time horizons and economic states. This provides a tremendous advantage to older economic modeling approaches, which relied on key assumptions to model the economy that often would not hold. Additionally, changing factors in the model based on a point in time would have been very difficult with this linear approach. With the ACE approach, economists can model an ever-changing economic environment dynamically, allowing to model the real-world more accurately than ever before.
Human-AI Augmentation is Human Replacement
One of the key components to the argument of AI enthusiasts is how it compares to other technological revolutions that spurred job creation. However, this is not always the case. As seen with the Luddites centuries ago, automation improving individual worker productivity does not mean that companies will simply hire more workers and produce more. Instead, firms are more likely to make decisions based on income and substitution effects. As a result, human-AI augmentation in the labor force will, over the long run, lead to a reinvestment by firms into AI and away from human labor. The scale of this is not yet apparent, but it could be significant, with some researchers estimating that some 30% of the workforce could be replaced with new-era AI automation.
Government Must Act
As was the case with manufacturing decline in the 2000's due to automation and off-shoring, government action through policy intervention will likely be required in order to optimize for social surplus. In this case, automation taxes may be helpful in slowing the transition to an AI-first labor force. Other programs, similar to Trade Adjustment Assistance programs in the late 2000's that helped the midwest, could serve a complementary role. Specifically, re-education and training programs will be required, and fiscal stimulus may also help in smoothing out the social losses spurred by increased unemployment.
Opportunities for Entrepreneurs
An interesting observation from our modeling was the emergence of companies in the model who offered non-AI products. This happened some time after AI was introduced as a factor of production, and replicates similar societal trends in the advance of technology, for example the surge in vinyl record sales following the advent of streaming.